본 내용은 Anaconda 와 Jupyter Notebook / Lab 을 이용하였습니다.
구현 환경이 설정되지 않으셨다면 아래 링크를 클릭하셔서 환경을 설치해줍니다.
모델 구현이 목적입니다. 실제로 어떻게 수정하여 쓰느냐에 따라 적용할 수 있는 방법입니다.
파이토치(PyTorch)
파이토치(PyTorch)는 2017년 초에 공개된 딥러닝 프레임워크로 개발자들과 연구자들이 쉽게 GPU를 활용하여 인공 신경망 모델을 만들고 학습시킬 수 있게 도와 줍니다. 파이토치의 전신이라고 할 수 있는 토치(Torch)는 루아 프로그래밍 언어로 되어 있었지만, 파이토치는 파이썬으로 작성되어 파이썬의 언어 특징을 많이 가지고 있습니다. 파이토치는 페이스북의 인공지능 연구팀(AI Research) 멤버들이 주로 관리하며, 독자적으로 운영되는 파이토치 포럼은 사람들이 질문을 올리면 프레임워크 개발자를 비롯한 많은 사람이 답을 해주는 등 활발히 교류가 일어나고 있습니다.
파이썬(Python)을 기반으로 하는 Scientific Computing 패키기지며 크게 2가지 목적을 가집니다.
- GPU를 제대로 이용하기 위한 NumPy의 대체제로 사용
- 최고의 유연성과 스피드를 제공하는 딥 러닝 연구 플랫폼
PyTorch
An open source deep learning platform that provides a seamless path from research prototyping to production deployment.
pytorch.org
먼저 설치하는 법에 대하여 알아보겠습니다.
설치하기
위 사이트로 들어가셔서 위의 상단 메뉴 중 Get Started 를 들어가줍니다.
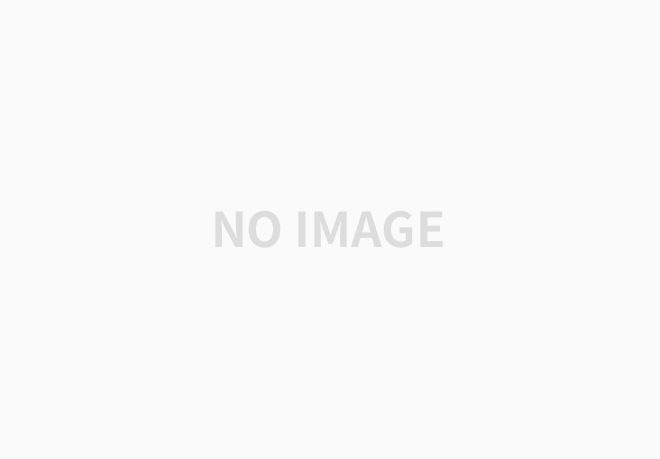
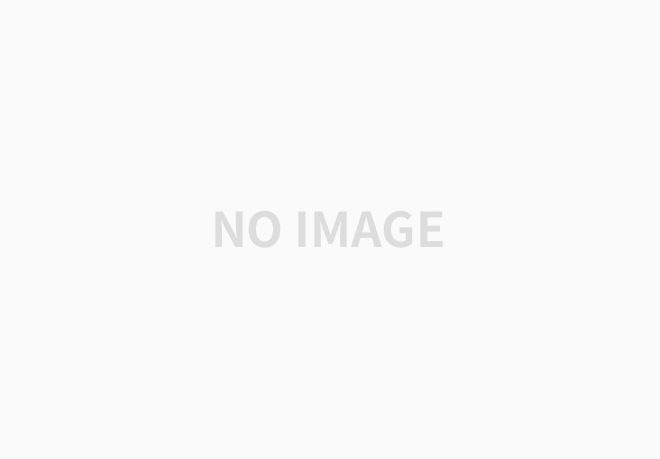
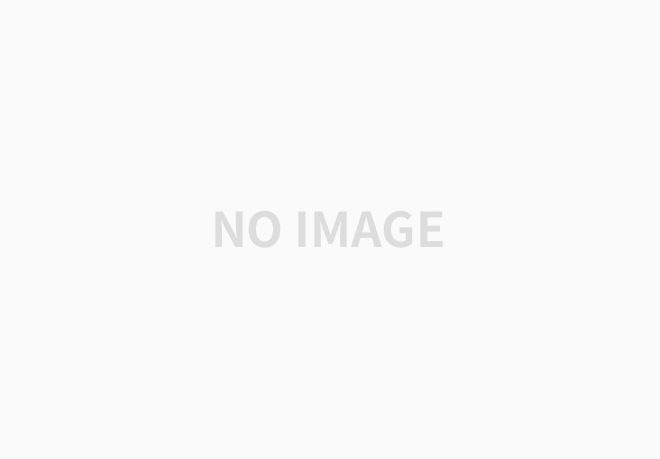
그리고 복사한 코드를 입력해줍니다.
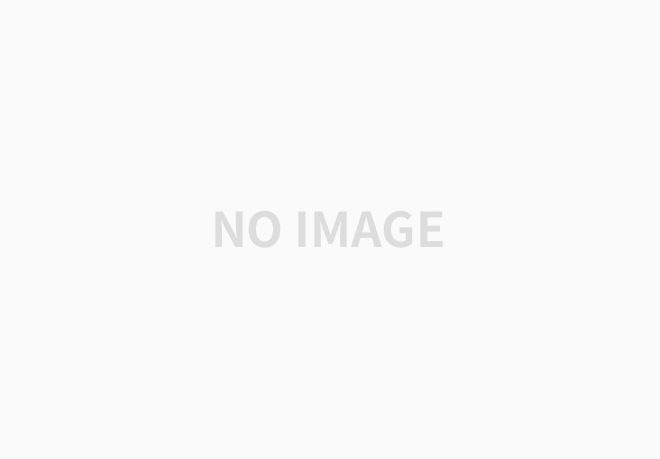
시작하기
텐서 (Tensors)
- 텐서는 파이토치의 가장 기본이 되는 데이터 구조와 기능을 제공하는 다차원 배열을 처리하기 위한 데이터 구조입니다.
- API 형태는 Numpy의 ndarray와 비슷하며 GPU를 사용하는 계산도 지원합니다.
- 텐서는 각 데이터 형태별로 정의되어 있습니다.
- torch.FloatTensor : 32bit float point
- torch.LongTensor : 64bit signed integer
- GPU 상에서 계산할 때에는 torch.cuda.FloatTensor를 사용합니다. (일반적으로 Tensor는 FloatTensor)
- 어떤 형태의 텐서이건 torch.tensor라는 함수로 작성할 수 있습니다.
텐서는 다양한 형식을 포함합니다.
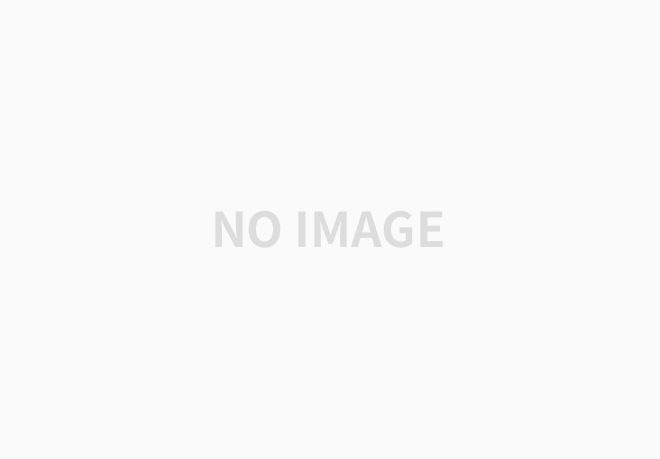
tensor를 정의하는 방법
'''
텐서 정의
'''
#지정텐서 정의
torch.tensor([[1., -1.], [1., -1.]])
>>> tensor([[ 1.0000, -1.0000],
[ 1.0000, -1.0000]])
torch.tensor(np.array([[1, 2, 3], [4, 5, 6]]))
>>> tensor([[ 1, 2, 3],
[ 4, 5, 6]])
tensor = torch.ones((2,), dtype=torch.int8)
data = [[0, 1], [2, 3]]
tensor.new_tensor(data)
>>> tensor([[ 0, 1],
[ 2, 3]], dtype=torch.int8)
# new_full(size, fill_value, dtype=None, device=None, requires_grad=False) → Tensor
# 사이즈에 지정값으로 채워진 텐서를 반환합니다.
tensor = torch.ones((2,), dtype=torch.float64)
tensor.new_full((3, 4), 3.141592)
>>> tensor([[ 3.1416, 3.1416, 3.1416, 3.1416],
[ 3.1416, 3.1416, 3.1416, 3.1416],
[ 3.1416, 3.1416, 3.1416, 3.1416]], dtype=torch.float64)
# new_empty(size, dtype=None, device=None, requires_grad=False) → Tensor
# 사이즈에 초기화되지 않은 데이터로 채워진 크기의 텐서를 반환합니다.
tensor = torch.ones(())
tensor.new_empty((2, 3))
>>> tensor([[ 5.8182e-18, 4.5765e-41, -1.0545e+30],
[ 3.0949e-41, 4.4842e-44, 0.0000e+00]])
# new_ones(size, dtype=None, device=None, requires_grad=False) → Tensor
# 사이즈에 1로 채워진 텐서를 반환합니다.
tensor = torch.tensor((), dtype=torch.int32)
tensor.new_ones((2, 3))
>>> tensor([[ 1, 1, 1],
[ 1, 1, 1]], dtype=torch.int32)
# 특정 데이터 유형의 텐서 정의
# torch.dtype 또는 torch.device 를 추가하여 텐서를 정의할 수 있습니다.
torch.zeros([2, 4], dtype=torch.int32)
>>> tensor([[ 0, 0, 0, 0],
[ 0, 0, 0, 0]], dtype=torch.int32)
cuda0 = torch.device('cuda:0')
torch.ones([2, 4], dtype=torch.float64, device=cuda0)
>>> tensor([[ 1.0000, 1.0000, 1.0000, 1.0000],
[ 1.0000, 1.0000, 1.0000, 1.0000]], dtype=torch.float64, device='cuda:0')
tensor 슬라이싱 및 접근
x = torch.tensor([[1, 2, 3], [4, 5, 6]])
print(x[1][2])
>>> tensor(6)
x[0][1] = 8
print(x)
>>> tensor([[ 1, 8, 3],
[ 4, 5, 6]])
torch.Tensor.item() 단일 값을포함하는 텐서에서 파이썬 숫자를 얻는데 사용
x = torch.tensor([[1]])
x
>>> tensor([[ 1]])
x.item()
>>> 1
x = torch.tensor(2.5)
x
>>> tensor(2.5000)
x.item()
>>> 2.5
그라디언트 자동 계산이 가능합니다. ( requires_grad=True, torch.autograd)
x = torch.tensor([[1., -1.], [1., 1.]], requires_grad=True)
out = x.pow(2).sum()
out.backward()
x.grad
>>> tensor([[ 2.0000, -2.0000],
[ 2.0000, 2.0000]])
기타 다른 torch.tensor를 보려면 https://pytorch.org/docs/stable/tensors.html에서 확인할 수 있습니다.
torch.Tensor — PyTorch master documentation
Shortcuts
pytorch.org
패션 MNIST 데이터 셋
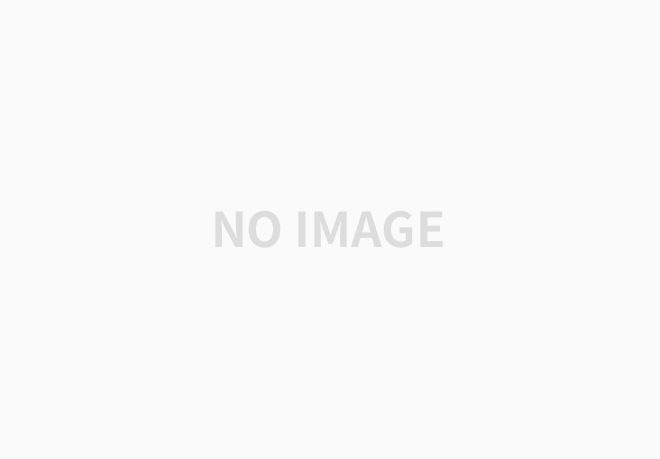
Fashion-MNISTZalando 의 기사 이미지에 대한 데이터 세트입니다. 60,000 개의 학습 세트와 10,000 개의 테스트 세트로 구성되어 있습니다. 각 예제는 28 개의 28 개의 회색조 이미지이며 10 개의 클래스 레이블과 연결되어 있습니다. 벤치마킹 머신 러닝 알고리즘을 위해 원래의 MNIST 데이터 세트 를 Fashion-MNIST 직접 대체 하는 역할을합니다 . 동일한 이미지 크기와 훈련 및 테스트 분할의 구조를 공유합니다. 의 제작자에 따르면 MNIST 데이터 세트를 대체해야 할 몇 가지 이유가 있습니다.Fashion-MNIST
- 데이터 세트 다운로드 (Download dataset (Using Dataloader))
- 데이터 세트 시각화 (Visualize dataset)
- LeNet
- GPU 장치 설정 (Setting the GPU device)
- LeNet 교육 및 평가 (Train and Evaluate LeNet)
- 시각화 손실 도표 (Visualization Loss Plot)
- Where to go from here?
- Conclusion
데이터 다운로드 : https://www.kaggle.com/
Kaggle: Your Machine Learning and Data Science Community
Kaggle is the world’s largest data science community with powerful tools and resources to help you achieve your data science goals.
www.kaggle.com
Google LLC의 자회사인 Kaggle은 데이터 과학자 및 머신 러닝 전문가로 구성된 온라인 커뮤니티입니다. Kaggle을 통해 사용자는 데이터 세트를 찾아서 게시하고, 웹 기반 데이터 과학 환경에서 모델을 탐색 및 구축하고, 다른 데이터 과학자 및 기계 학습 엔지니어와 협력하고, 데이터 과학 과제를 해결하기 위해 경쟁에 참여할 수 있습니다.
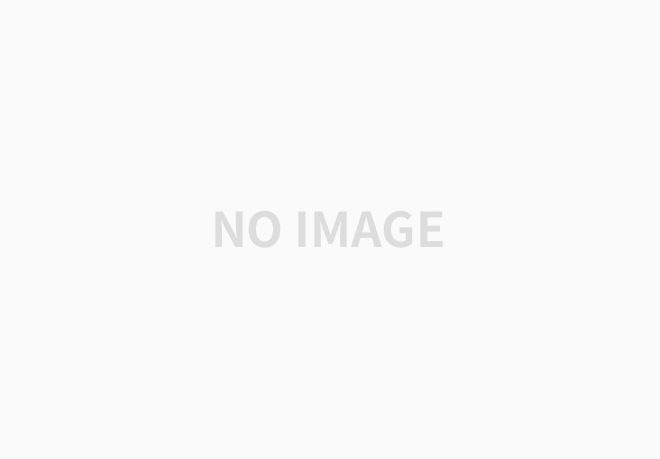
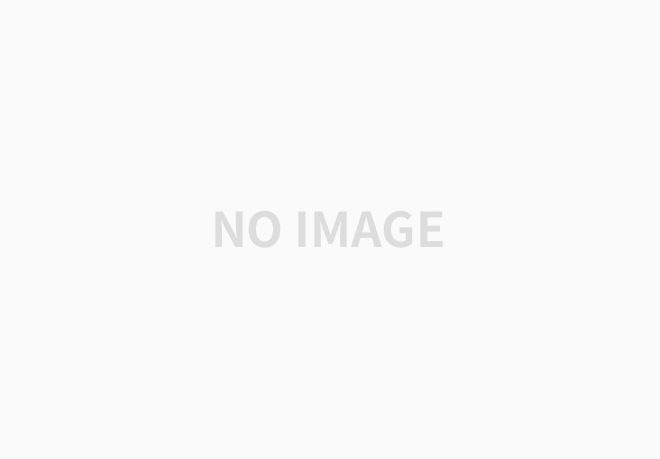
200MB 라고 쓰여져 있는데 용량은 다를 수 있습니다.
데이터를 다운로드 받고 난 후 압축을 풀어줍니다.
데이터 세트 불러오기
코드는 Jupyter Lab을 이용하여 구현하였습니다.
데이터를 불러오기 앞서 필요한 라이브러리를 설치합니다.
- matplotlib
- pandas
!conda install matplotlib -y
!conda install pandas-y
데이터 불러오기 코드
from torch.utils.data import Dataset, DataLoader
import pandas as pd
class FashionMNISTDataset(Dataset):
def __init__(self, file_path, transform=None):
self.transform = transform
self.data = []
df_mnist = pd.read_csv(file_path)
for idx in df_mnist.index:
temp_input = df_mnist.values[idx][1:]
temp_target = df_mnist.values[idx][0]
self.data.append((temp_input, temp_target))
def __len__(self):
return len(self.data)
def __getitem__(self, idx):
# 해당 셀에 데이터를 가져온다.
x, y = self.data[idx]
# 튜플 형식으로 지정해준다.
sample = {"x" : x, "y" : int(y)}
if self.transform:
sample = self.transform(sample)
return sample
train_dataset = FashionMNISTDataset("/압축해제한 폴더명/fashion-mnist_train.csv")
test_dataset = FashionMNISTDataset("/압축해제한 폴더명/fashion-mnist_test.csv")
# 리스트는 그 값의 생성, 삭제, 수정이 가능하지만 튜플은 그 값을 바꿀 수 없다.
# train_dataset[0]["y"] = 3
# train_dataset[0]["y"]
#>>> 2
불러온 데이터 확인 코드
import numpy as np
import matplotlib.pyplot as plt
print(train_dataset[0]["y"])
# 데이터에 저장된 이미지는 1차원으로 길게 list 형식으로 되어 있다.
# 그렇기 때문에 reshape를 이용하여 원래의 사이즈로 맞춰준다.
plt.imshow(train_dataset[0]["x"].reshape([28, 28]))
plt.show()
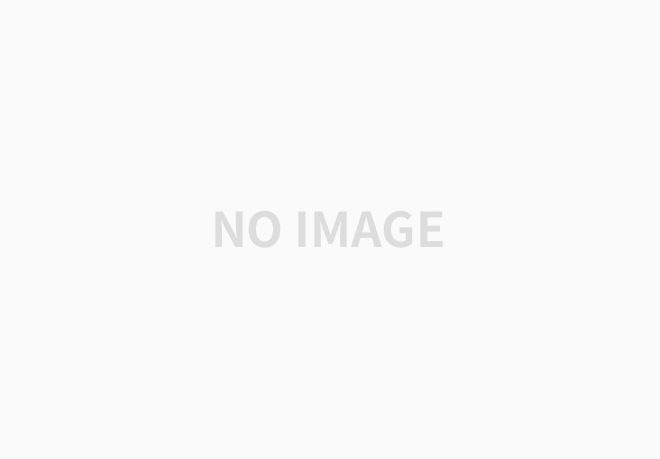
모델 구현 코드
import torch.nn as nn
import torch.nn.functional as F
class MyModel(nn.Module):
def __init__(self):
super(MyModel, self).__init__()
self.model = nn.Sequential(nn.Linear(784,512),
nn.ReLU(),
nn.Linear(512,256),
nn.ReLU(),
nn.Linear(256,128),
nn.ReLU(),
nn.Linear(128,64),
nn.ReLU(),
nn.Linear(64,10))
def forward(self, inputs):
x = self.model(inputs)
outputs = F.log_softmax(x, dim = 1)
return(outputs)
model = MyModel()
print(model)
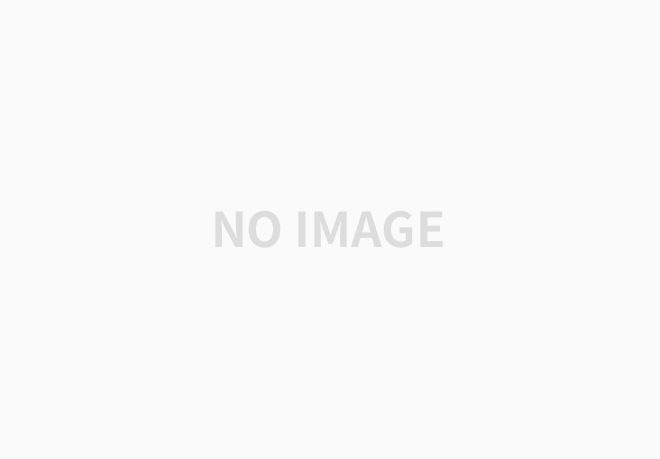
테스트 구현 코드
def test_code(_test_dataloader):
test_loss = 0
correct = 0
count = 0
with torch.no_grad():
for n, sample in enumerate(_test_dataloader):
outputs = model(sample['x'].float())
preds = outputs.argmax(dim=1, keepdim=True)
correct += preds.eq(sample["y"].view_as(preds)).sum().item()
loss = F.nll_loss(outputs, sample['y'], reduction="sum").item()
test_loss += loss
count += n
test_loss /= count
accuracy = correct / count
return test_loss, accuracy
모델 트레이닝 및 테스트 코드
import torch.optim as optim
train_dataloader = DataLoader(train_dataset, batch_size=8, num_workers=2, shuffle=True)
test_dataloader = DataLoader(test_dataset, batch_size=8, num_workers=2, shuffle=True)
optimizer = optim.Adam(model.parameters(), lr=0.001)
criterion = nn.NLLLoss()
loss_arr = []
loss_epoch_arr = []
epochs = 11
for epoch in range(epochs):
print("epoch : ", epoch, "/", epochs)
for n, sample in enumerate(train_dataloader):
outputs = model(sample['x'].float())
loss = criterion(outputs, sample['y'])
if n % 500 == 0:
print("{}th: loss: {:.4f}".format(n, loss))
optimizer.zero_grad()
loss.backward()
optimizer.step()
loss_arr.append(loss.item())
loss_epoch_arr.append(loss.item())
if epoch % 1 == 0:
test_loss, accuracy = test_code(test_dataloader)
print("test_loss : {:.4f} , accuracy : {:.4f}".format(test_loss, accuracy))
결과 화면
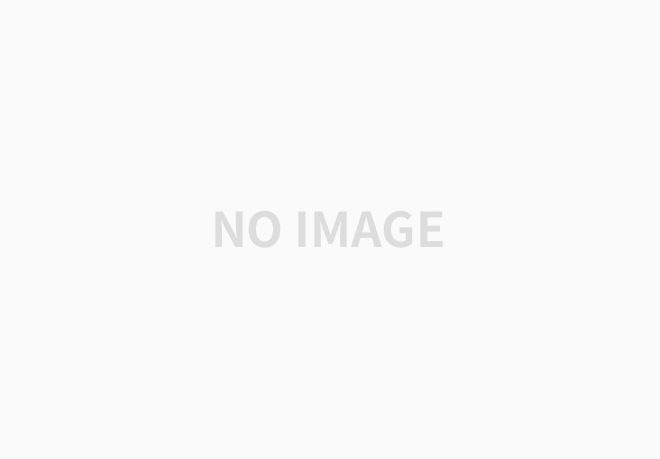
그래프 확인
plt.plot(loss_epoch_arr)
plt.xlabel("Epoch")
plt.ylabel("Loss")
plt.show()
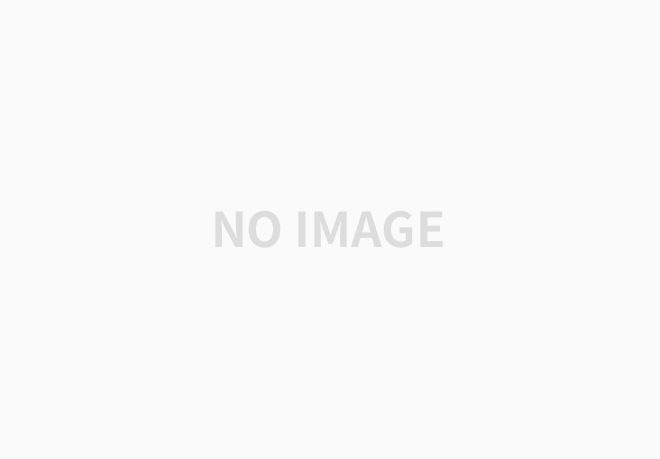
따로 어떻게 하겠다 정하지 않고 구현하였기 때문에 Loss가 이렇게 나올 수 밖에 없다.
구현이 목적
'Programming > (Python)(Ubuntu)' 카테고리의 다른 글
Seq2Seq 알고리즘 이해 + Attention (1) | 2020.02.27 |
---|---|
PyTorch Dataset Class, transform 설명 (0) | 2020.02.20 |
우분투 vi 에디터 사용법 (0) | 2020.02.15 |
Sentence piece 를 이용한 문장 분류기 만들기 (1) | 2020.02.14 |
구글: SentencePiece 사용법 Google: SentencePiece Tutorial (1) | 2020.02.14 |